Natural language processing (NLP), is the most indispensable part of AI and has already transformed the way we communicate with the external world. Technically speaking, it uses computational and mathematical methods to analyze the human language to facilitate interactions with machines using conversational language.
“According to a study by Tractia, the market opportunity for NLP is expected to grow to $22.3 billion by the end of 2025.”
In simple words, it is the technology that allows humans to communicate with machines. In many contexts and applications, it is also known as computational linguistics combines technologies like Artificial Intelligence (AI), Machine Learning (ML), and the natural language of humans.
Without a doubt, NLP has served as an effective communication bridge between humans and machines. So if you were imagining the phrases like Hey Siri, or Hey Alexa and tell you about the nearest coffee shop. It is the complex algorithm of NLP that allows Siri, Alexa, or Voice interface to respond
And with the implementation of semantic search, which is true, an advanced version of keyword-based search. It is possible for the search engines to learn associations between the words and understand the context and meaning of the query to provide the right response. Fortunately, you are witnessing this turnaround because of the NLP technology.
Yes, semantic research is a part and parcel of NLP. But over the years, NLP has been transformed into a better and efficient version of it. So to provide more clarity on NLP, here we are, presenting some significant improvements over the years.
The improvement is not only about the increasing amount of data, but NLP models have improved a lot in terms of accuracy and scalability. But some advancements will decide the future of NLP and its applications. Here they are:
- Implementation of BERT: If you don’t know about BERT, it is Bidirectional Encoder Representations from Transformers, and a new and open-source pre-training model. The bidirectional approach of the BERT model allows a model to not only read left to right but allow the model to get the entire context of the sentence. And it is very simple to apply. A developer with a good sense of mind can customize the pre-trained models to be more accurate. To be more precise, it is somehow similar to learning the meaning of a sentence, when we don’t focus on the meaning of a particular word but the whole sentence. In this case, we subconsciously read ahead to better understand the border sentence.
- Summarization and interfacing : Most the customers want a model that extracts the key info from a big paragraph or document and has the ability to deliver a crisp and lucid summary. More precisely, users want to ask a question and have the model infer the answer. Now, chatbots run on NLP are in process to deliver on this requirement.
- Sentiment and emotion detection accuracy: NLP is known for its emotion and sentiment detection ability. But its ability within a piece of text still needs to be improved. Deep sentiment analysis is very complex, especially the views expressed on social media or in op-eds. Talking about sentiments can be anger, joy, sadness, hesitation, confidence, and indifference. And it is very typical to identify the one with a high degree of confidence. But a custom NLP model helps to detect these nuances.
Now, it comes to how NLP functions and what prospects it brings on the table:
NLP makes understanding human emotions easier
It has been discussed that NLP has the ability to extract valuable insights from human texts and understand complex human emotions. For instance: if someone says the good is delicious, NLP will show human emotion is happiness at present. Going further, it can also differentiate between complex emotions like sad, joy, anger, annoyed, and more. This type of understanding has a typical name termed as sentimental analysis.
And it is well known that emotions influence consumer choices, so it is quintessential to track emotions to serve customers with an enhanced experience. Sentiments can be derived from various queries and their answers, reviews, and feedback given by the customers on multiple social media channels, web pages, and sites.
NLP helps in the categorization of data
As an efficient data technology, NLP has the ability to understand the data and categorize it into multiple data sets. For instance: Data extraction includes entity extraction (names, demographic, certain keywords), categorization of text (emotions, different categories, by context), data clustering (according to topics new and old), facts (validated and structured information for better analysis), relationship extraction, (helps in establishing real-world relationships through texts).
And NLP can put different components of data that can be collected and put into a structured format to use in marketing campaigns.
NLP helps in filtering spam emails from the mailbox
Email marketing has always been a great tool in reaching out to the target audiences. And NLP can be a great use in email filters. It helps in filtering the spam messages from the servers and separates them before it reaches the mailboxes.
NLP recognized the human voice
By making use of NLP and AI technology, companies can create voice interfaces and Chatbots that can talk with customers anytime and every time. These voice interfaces have the ability to understand customers’ voices, recognize the words and sentiments, and reply to their queries with appropriate solutions. NLP can convert voice/speech into text helping machines to understand the human language easily.
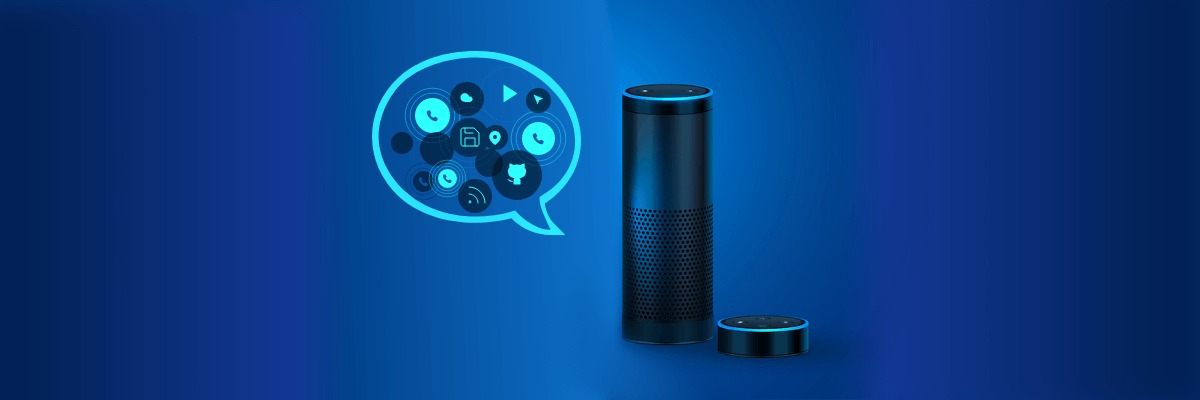
“The chatbot industry is expected to grow past $10 billion by 2026”.
NLP offers more personalized customer service
Customer service is one of the key areas of a successful business. Companies that take efforts to improve customer service and offer more personalized services to their customers flourish more in the market. Voice recordings, text messages, and online responses can be collected through the systems and analyzed for deeper insights, further providing ways to offer tailor-made services to the customers.
NLP offers targeted ads and marketing campaigns
Brand advertising is quite compulsory for any brand to make it high in the market. But it is equally important to reach the right audience at the right time through the right channel. Here, NLP identifies consumer behavior and choices through sources like Social media pages, keyword searches, online responses, emails, purchasing patterns, and others.
In the process, NLP identifies the data, categorizes it into different buyer personas, and empowers marketers to customize ad campaigns specific to the personas. By following this way, organizations can target customers in a better way with a more personalized experience. In the most precise words, it performs keywords in the most accessible format.
NLP helps in reputation monitoring
NLP deals in big data and by analyzing that data, it helps organizations to stay updated with the industry trends through its in-built market intelligence ability. It includes insights from competitors, stakeholders, governments, and other regulatory bodies.
NLP helps in tracking these market reports which are very significant by the way for financial organizations that have an eye on changing numbers, profits, loss, and market status.
Human resource management
It may not be a real concern for many, but organizations need it to secure talent to work for them. Here NLP steps in and helps in resume segregation that makes HR’s life easier, determining spam, regulatory compliance, biometrics, robotics, etc. NLP is gaining importance in business activities slowly, and companies are investing majorly into the technology.
Although there is more research work required and the technology is still evolving, NLP is undoubtedly helping businesses to stay ahead in the competition proving to be the future of every business.
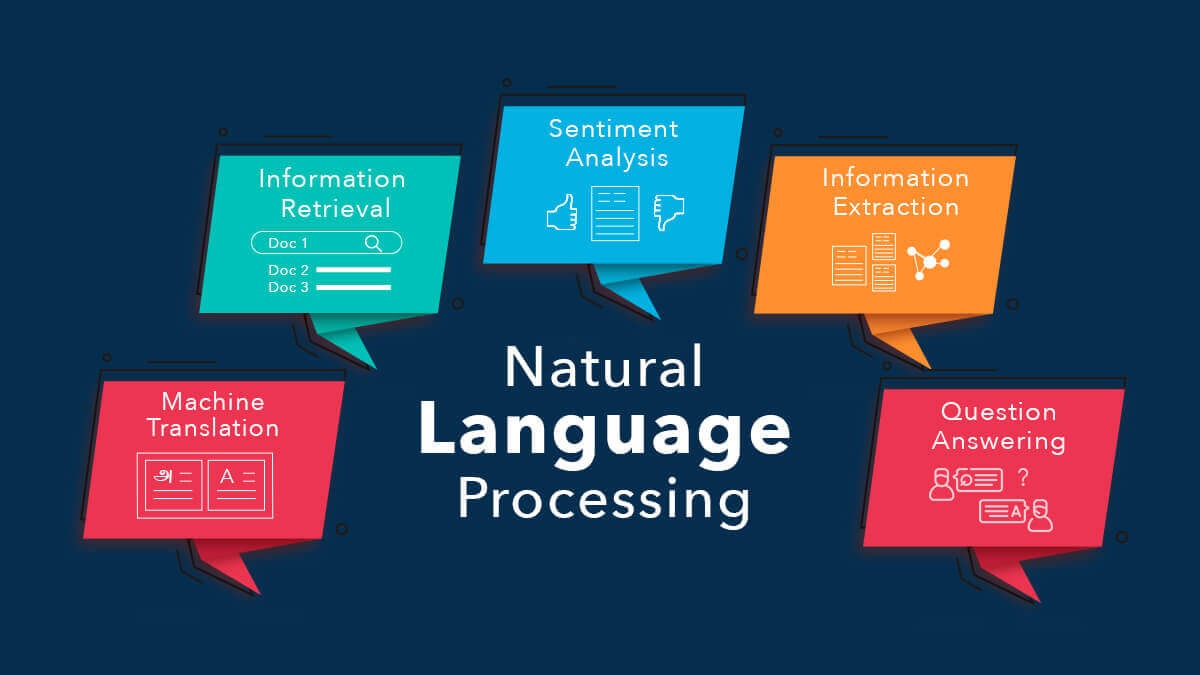
Bringing it all together
Now you have already understand that the terms, speech to text, visual recognition, and text analytics come under the big umbrella of NLP. These three fields of AI are on a massive upswing and will eventually serve as a singular function. And this is not the end, NLP is set and much advanced to play a significant role in the AI landscape through computational power, scalability, and of course, from the current digital wave.
However, NLP’s outcomes will only be as good as the data pipelines built underneath to support the models for greater training, detection, summarization, and accuracy.